What is customer sentiment analysis? It’s a way to measure how customers feel about your business, products, or services by analyzing their interactions, feedback, and sentiments. This can be done using sentiment analysis tools that process unstructured data from sources like survey responses, social media posts, and customer support interactions.
But why should businesses care about tracking customer sentiment? Because understanding customer sentiment is a game-changer for enhancing customer satisfaction, loyalty, and overall experience. By analyzing customer sentiment, businesses can detect negative sentiment, address pain points, and turn unhappy customers into satisfied customers.
In this guide, we’ll dive into the details of customer sentiment analysis, from how it works to the tools and technologies that make it possible. You’ll learn how to measure customer sentiment, gain actionable insights, and improve customer interactions at every touchpoint.
What is Customer Sentiment Analysis?
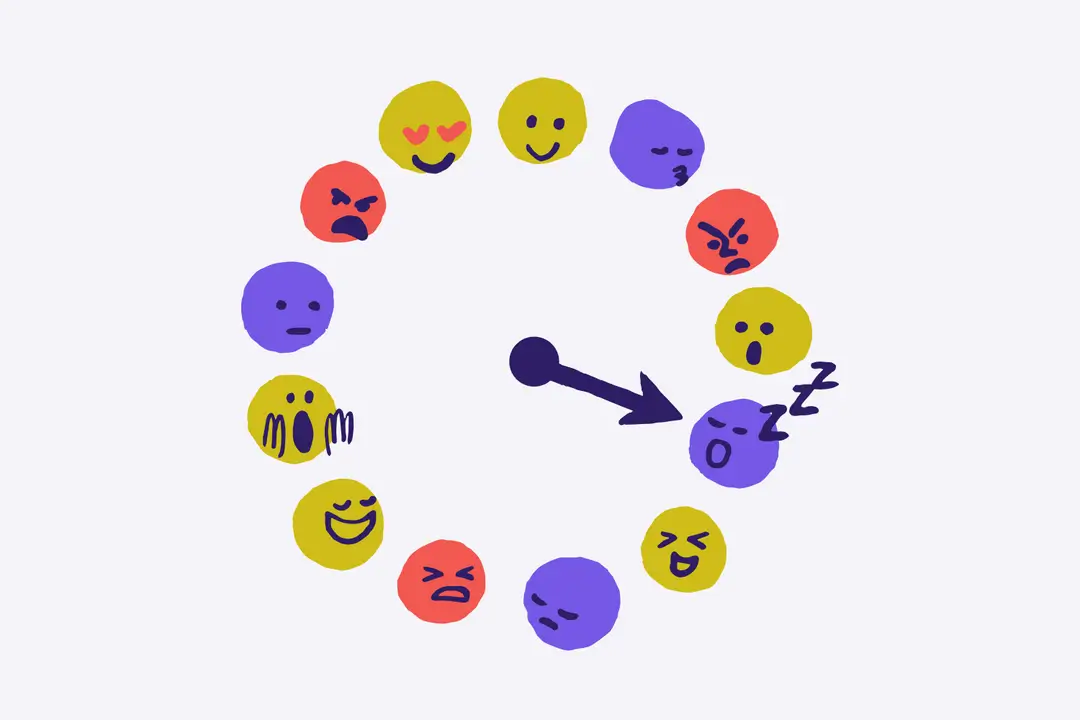
Customer sentiment analysis is the process of evaluating customer feedback to determine whether it reflects positive, negative, or neutral sentiments. By analyzing customer sentiment, businesses gain valuable insights into how customers feel about their brand, products, or services.
This technique leverages natural language processing (NLP), machine learning, and other sentiment analysis technologies to analyze unstructured data like survey responses, social media comments, and customer service chats. The goal is to extract sentiment insights that can inform decisions, improve customer experience, and enhance customer satisfaction.
How Does Sentiment Analysis Work?
The process typically involves:
- Data Collection: Gathering data points from sources like social media monitoring, feedback forms, direct feedback, and customer service interactions.
- Text Analysis: Using sentiment analysis tools powered by natural language understanding to detect emotions and classify text as positive, negative, or neutral.
- Scoring Sentiment: Assigning a customer sentiment score to each interaction, helping businesses measure customer sentiment quantitatively.
- Generating Insights: Providing actionable insights based on trends, like identifying areas where customers express frustration or pain points that need addressing.
Types of Sentiment Analysis
- Aspect-Based Sentiment Analysis: Focuses on specific aspects of a product or service. For example, a customer might love the product quality but express dissatisfaction with the shipping process.
- Multilingual Sentiment Analysis: Analyzes feedback in multiple languages to reach a broader audience.
- Social Media Sentiment Analysis: Tracks social media posts and comments to understand how customers react to marketing campaigns or product launches.
By employing these methods, businesses can dive deeper into customer sentiment data, enabling them to predict behaviors like customer churn and ensure more satisfied customers.
Why is Sentiment Analysis Important?
Sentiment analysis is important because it helps businesses understand how customers feel about their brand, products, and services. This understanding is the foundation for making informed decisions that can enhance customer satisfaction, loyalty, and overall experience.
Benefits of Sentiment Analysis
Improved Customer Experience
Tracking customer sentiment allows businesses to identify pain points in customer interactions. This leads to actionable changes that create a smoother experience for customers, making them feel valued and understood.
Enhanced Customer Satisfaction
Analyzing survey responses, feedback forms, and customer service chats provides businesses with insight into what satisfies their customers. By addressing these areas, businesses can create more satisfied customers and improve customer loyalty.
Early Detection of Negative Sentiment
Sentiment analysis tools detect negative sentiment early in the customer journey. For example, monitoring social media comments can help businesses address complaints before they escalate into public relations issues.
Boosted Marketing Campaigns
Insights from social media monitoring tools reveal how customers react to marketing campaigns. Businesses can refine their strategies based on these insights to improve engagement and outcomes.
Customer Retention and Loyalty
Businesses that measure customer sentiment effectively can reduce customer churn. Understanding the feelings customers have about their experiences allows businesses to make proactive changes, increasing the likelihood of repeat customers.
Data-Driven Decision Making
By analyzing customer sentiment data, businesses gain a deeper understanding of trends and behaviors. This data becomes a powerful tool for shaping business strategies and meeting customer expectations.
Real-World Applications
- E-Commerce Businesses: Track how customers react to new product launches through their reviews and feedback.
- Customer Support Teams: Analyze customer support interactions to identify common complaints and improve processes.
- SaaS Companies: Monitor usage feedback to enhance product functionality and address user pain points.
Why Businesses Can’t Ignore Sentiment Analysis
In today’s competitive market, businesses must be proactive in understanding how customers feel. Ignoring this can lead to missed opportunities, dissatisfied customers, and increased churn. Sentiment analysis provides the sentiment insights needed to stay ahead and maintain a positive brand reputation.
How Does Sentiment Analysis Work?
The process of sentiment analysis involves several steps to extract, analyze, and interpret customer sentiment data. By understanding these steps, businesses can implement sentiment analysis effectively to improve customer satisfaction and loyalty.
Key Steps in Sentiment Analysis
Data Collection
Sentiment analysis begins with gathering data points from various sources:
- Social media posts and comments
- Customer feedback through surveys and feedback forms
- Customer service chats and email interactions
- Direct feedback from customer reviews and testimonials
Data Preprocessing
Before analyzing the data, it’s cleaned and prepared. This step removes irrelevant information, corrects spelling errors, and processes unstructured data. Preprocessing ensures that the data is ready for sentiment analysis tools to interpret.
Natural Language Processing (NLP)
Sentiment analysis uses natural language processing (NLP) to break down text into understandable components. This includes:
- Tokenization (breaking text into words or phrases)
- Part-of-speech tagging
- Recognizing expressions like sarcasm or irony
Sentiment Classification
Text is classified into categories like positive sentiment, negative sentiment, or neutral sentiment. Tools like sentiment analysis software rely on machine learning models and predefined rules to make these classifications.
Sentiment Scoring
Each piece of feedback is assigned a sentiment score, which quantifies the intensity of the sentiment. For example:
- A score close to +1 indicates very positive sentiment.
- A score near -1 represents strong negative sentiment.
- Neutral feedback receives a score close to 0.
Analyzing Context
Advanced techniques like aspect-based sentiment analysis identify specific parts of the feedback. For instance, a review may be positive about customer service but negative about the product’s quality.
Example of Sentiment Analysis in Action
Let’s say a customer leaves the following review: “The product quality was excellent, but the delivery was delayed.”
- Aspect-Based Sentiment Analysis: The product quality receives a positive sentiment score, while the delivery process receives a negative sentiment score.
- Actionable Insights: Focus on improving logistics while maintaining product standards.
Tools That Make Sentiment Analysis Possible
There are numerous sentiment analysis tools and technologies available to automate this process. These tools use artificial intelligence to ensure accurate and scalable sentiment analysis across large datasets.
Sentiment Analysis Tools and Technologies
To successfully implement sentiment analysis, businesses need reliable tools and technologies that can handle vast amounts of customer data and provide actionable insights. These tools range from simple software solutions to advanced platforms powered by artificial intelligence and natural language processing (NLP).
Popular Sentiment Analysis Tools
Lexalytics
- An NLP-driven tool that provides sentiment insights from text data.
- Ideal for analyzing social media posts, survey responses, and customer service chats.
- Offers multilingual sentiment analysis capabilities.
MonkeyLearn
- A versatile platform for performing aspect-based sentiment analysis.
- Supports custom machine learning models for more precise sentiment scores.
- Integrates with tools like Slack and Google Sheets for seamless workflows.
Hootsuite Insights
- Specializes in social media monitoring and analyzing customer emotions.
- Detects customer sentiment in real-time to track campaign performance.
- Great for identifying positive, negative, or neutral sentiments across platforms.
IBM Watson Natural Language Understanding
- Uses natural language understanding (NLU) to classify and analyze text.
- Supports detecting customer opinions and classifying unstructured data into actionable categories.
- Excels in tracking customer interactions and trends.
Google Cloud Natural Language API
- Leverages Google’s AI capabilities for accurate sentiment analysis.
- Designed to process large datasets, such as feedback from customer satisfaction surveys or social media comments.
- Offers insights that help businesses enhance customer expectations.
Key Features to Look For
When selecting a sentiment analysis software, consider these features to ensure it meets your needs:
- Multilingual Support: Essential for businesses with a global customer base.
- Real-Time Analysis: Enables faster responses to customer feedback and issues.
- Integration Capabilities: Should work seamlessly with CRM systems, survey tools, and other platforms.
- Scalability: Must handle large volumes of data, especially for enterprises or businesses managing social media monitoring.
How to Choose the Right Tool
To select the best tool for your business, consider:
- Type of Data: Are you analyzing customer service interactions, marketing campaigns, or survey responses?
- Team Size: Small businesses and startups might prefer cost-effective options, while larger enterprises may need robust tools with advanced features.
- Customization Needs: Some businesses require sentiment analysis models tailored to specific industries or customer pain points.
Future of Sentiment Analysis Technologies
Emerging technologies like machine learning and natural language understanding continue to improve sentiment analysis accuracy. For example:
- AI-Powered Models: These models can analyze complex sentences, including those with sarcasm or mixed emotions.
- Advanced Sentiment Metrics: Tracking sentiment over time to predict trends like customer churn or repeat customers.
By leveraging the right tools and technologies, businesses can gain actionable insights, improve customer sentiment, and create more satisfied customers.
Best Practices for Implementing Sentiment Analysis
Implementing sentiment analysis effectively requires a clear strategy, the right tools, and a focus on actionable outcomes. These best practices will help businesses harness sentiment insights to enhance customer satisfaction and loyalty.
1. Define Clear Objectives
- Identify what you want to achieve. Are you aiming to improve customer experience, monitor marketing campaigns, or reduce customer churn?
- Align your sentiment analysis efforts with measurable business goals, like increasing repeat customers or enhancing customer loyalty.
2. Use Diverse Data Sources
- Gather data from multiple touchpoints, including:
- Customer feedback from surveys and forms
- Social media comments and posts
- Customer support interactions, such as chats and emails
- This provides a comprehensive view of how customers feel.
3. Leverage Aspect-Based Sentiment Analysis
- Focus on specific aspects of your business, like product quality, customer service, or delivery processes.
- For instance, if customers leave mixed feedback, isolate what’s causing positive sentiment and what’s driving negative sentiment.
4. Ensure Data Accuracy
- Preprocess data to remove irrelevant content, fix typos, and filter noise like spam or repeated entries.
- This ensures the sentiment analysis tools work with clean and relevant customer sentiment data.
5. Monitor Real-Time Sentiment
- Use social media monitoring tools to track brand mentions and detect negative sentiment quickly.
- Responding promptly to issues can help maintain customer trust and improve overall sentiment.
6. Integrate Sentiment Analysis with Business Processes
- Embed sentiment analysis insights into key areas like customer service, marketing, and product development.
- For example, share sentiment scores with your support team to help them address recurring customer pain points.
7. Train Your Sentiment Analysis Models
- If you’re using custom sentiment analysis models, train them regularly with new data to improve accuracy.
- Include complex scenarios like sarcasm, ambiguous phrases, and multilingual feedback.
8. Focus on Actionable Insights
- Prioritize insights that can lead to immediate changes, such as addressing complaints highlighted in direct feedback.
- Track how implementing these changes improves customer satisfaction surveys or reduces negative sentiment.
9. Regularly Evaluate Performance
- Measure the success of your sentiment analysis efforts by monitoring key metrics:
- Change in customer sentiment scores
- Reduction in customer issues and complaints
- Improvements in customer expectations and loyalty
- Adjust your strategy based on the results.
10. Collaborate Across Teams
- Share sentiment insights with all relevant teams, including customer support, marketing, and product development.
- A united effort ensures that everyone works towards improving how customers feel.
Example of Effective Implementation
An e-commerce company uses sentiment analysis software to track feedback from customer reviews and social media posts. By identifying complaints about delivery times, they make improvements and see a 15% increase in satisfied customers within three months.
Challenges in Sentiment Analysis and How to Overcome Them
While sentiment analysis offers significant benefits, businesses often encounter challenges in its implementation. Addressing these obstacles ensures that sentiment analysis delivers accurate and actionable insights.
1. Understanding Context and Nuance
- Challenge: Sentiment analysis tools may misinterpret nuances like sarcasm, humor, or cultural differences. For example, the phrase “Great, another delay!” could be classified as positive sentiment if not properly analyzed.
- Solution: Use advanced sentiment analysis technologies like those powered by natural language understanding (NLU) to recognize context. Incorporate machine learning models trained on diverse data sets to improve accuracy.
2. Handling Unstructured Data
- Challenge: Customer sentiment data often comes in unstructured formats, such as free-form survey responses, social media posts, or feedback forms, making it difficult to process.
- Solution: Use tools designed for processing unstructured data. These tools can extract sentiment from messy text formats and convert them into structured insights.
3. Detecting Mixed Sentiment
- Challenge: A single piece of feedback may contain mixed sentiment. For instance, “The product works well, but the support team was unresponsive.”
- Solution: Implement aspect-based sentiment analysis to break down feedback into specific components, enabling businesses to address positive and negative sentiments separately.
4. Multilingual Sentiment Analysis
- Challenge: Businesses that serve global customers need to analyze feedback in multiple languages, which can lead to inconsistencies.
- Solution: Use multilingual sentiment analysis tools to process feedback in various languages. Choose tools that support automatic translation or are trained in the target languages.
5. Data Volume and Scalability
- Challenge: Managing and analyzing large datasets, such as thousands of social media comments or survey responses, can be overwhelming.
- Solution: Opt for sentiment analysis software with robust scalability and real-time processing capabilities. Cloud-based tools like Google Cloud Natural Language API are great for handling high data volumes.
6. Accuracy and False Positives
- Challenge: Sentiment analysis models can misclassify text, leading to false positives or negatives, especially when dealing with complex customer feedback.
- Solution: Regularly update and train your sentiment analysis models with new data. Use hybrid approaches that combine machine learning with rule-based systems for improved accuracy.
7. Limited Actionability of Insights
- Challenge: Extracted insights may not always lead to actionable changes if not aligned with business priorities.
- Solution: Collaborate with relevant teams to connect insights with specific actions. For example, share insights about customer support interactions with the support team to address recurring issues.
Case Studies: Successful Sentiment Analysis in Action
Real-world examples demonstrate how businesses have used sentiment analysis to enhance customer satisfaction, improve loyalty, and make data-driven decisions. Let’s explore a few case studies showcasing its impact.
Case Study 1: E-Commerce Company Improves Customer Loyalty
Challenge:
An e-commerce business struggled with negative reviews related to shipping delays and inconsistent customer service. These issues were impacting customer loyalty and increasing customer churn.
Solution:
- Implemented sentiment analysis tools to process reviews, social media comments, and support tickets.
- Used aspect-based sentiment analysis to isolate issues related to shipping and customer service.
- Introduced real-time alerts for negative sentiment detection, enabling faster responses to complaints.
Outcome:
- Shipping processes were optimized, reducing delays by 40%.
- Customer satisfaction scores improved by 25%.
- The company achieved a 15% increase in repeat customers over six months.
Case Study 2: SaaS Company Enhances Customer Support
Challenge:
A SaaS company received inconsistent feedback about its software and support team. They needed to understand customer emotions in support interactions and product reviews.
Solution:
- Deployed sentiment analysis software integrated with their CRM system to track support tickets and chat logs.
- Used natural language processing (NLP) to detect sentiments in conversations.
- Trained their customer service team to address common pain points highlighted by negative feedback.
Outcome:
- Reduced response times for support tickets by 30%.
- Increased customer satisfaction survey scores by 20%.
- Gained actionable insights to guide product updates, resulting in better feedback.
Case Study 3: Retail Brand Monitors Marketing Campaigns
Challenge:
A retail brand needed to evaluate how customers responded to their new product line and marketing campaigns across various channels, including social media posts.
Solution:
- Used social media monitoring tools and sentiment analysis to track mentions and customer feedback.
- Identified key trends, such as positive sentiment for product quality but negative sentiment around pricing.
- Adjusted their pricing strategy and personalized campaigns to address customer concerns.
Outcome:
- Increased product sales by 18% in the next quarter.
- Improved overall brand perception, as reflected in sentiment scores.
- Enhanced the effectiveness of future marketing campaigns.
Conclusion
Customer sentiment analysis helps businesses understand how customers feel and take actions to improve their experiences. By using sentiment analysis tools, businesses can process data from social media posts, feedback forms, and support chats to identify negative sentiment, improve customer satisfaction, and reduce customer churn.
When businesses track and act on sentiment insights, they enhance customer loyalty, meet customer expectations, and build stronger relationships. Adopting sentiment analysis is a smart step toward staying competitive and driving growth.
Frequently Asked Questions
1. What is customer sentiment analysis?
Customer sentiment analysis is the process of evaluating customer feedback to determine if it reflects positive, negative, or neutral emotions. It helps businesses understand how customers feel about their brand, products, or services.
2. How does sentiment analysis work?
Sentiment analysis uses natural language processing (NLP) and machine learning to analyze text from sources like surveys, social media, and customer chats. It classifies text as positive, negative, or neutral and assigns sentiment scores to quantify emotions.
3. What is aspect-based sentiment analysis?
Aspect-based sentiment analysis focuses on specific elements of a product or service. For example, it can analyze if customers like product quality but dislike shipping services.
4. Can sentiment analysis handle multiple languages?
Yes, with multilingual sentiment analysis tools, businesses can analyze feedback in various languages, making it ideal for global audiences.